NVIDIA has set new standards for graphics cards with its recent advancements. Other than the graphics memory and speed, it has also worked on developing specialized cores for various tasks. These specialized cores revolutionized modern GPUs and unlocked new development areas. Examples of such cores include tensor, RT, and CUDA cores. So, it is important to understand these advanced features if you want to catch up with modern GPUs.
Key Takeaways
- Tensor cores are specialized in NVIDIA GPUs that excel at fast matrix manipulations, providing acceleration for deep learning workloads.
- DLSS technology effectively utilizes tensor cores to improve FPS count by leveraging AI techniques to generate additional frames.
- You must tailor your workloads into specialized workloads to maximize GPU utilization with tensor cores.
Tensor Cores
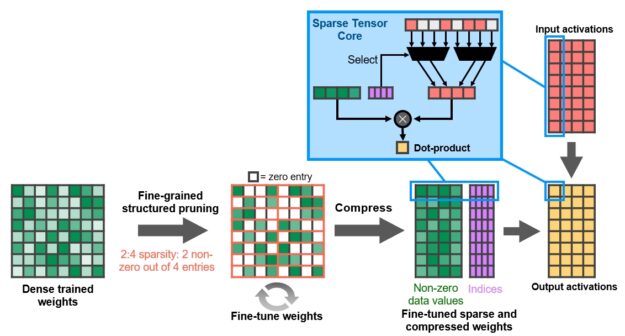
Tensor cores are specialized NVIDIA hardware units that are designated for matrix operations. A simpler way of understanding these cores is thinking of them as powerful calculators that handle complex mathematical operations[1]. Those complex mathematical operations are called tensor computations. Just like a regular calculator can perform basic arithmetic like addition and multiplication, tensor cores excel at performing more advanced calculations involving large sets of numbers.
Such calculations are often required in artificial intelligence, deep learning, and machine learning. So, these cores greatly accelerate the workflow in said fields by rapidly manipulating matrices. Through these cores, NVIDIA has expanded its target audience significantly. They are stepping up their game from gamers to data scientists, analysts, and deep learning experts[2].
Tensor Cores Vs. CUDA Cores Vs. RT Cores Vs. Compute Units
Below is a comprehensive overview of the two modern NVIDIA cores and how they compare to each other:
Tensor Cores | CUDA Cores | RT Cores | Compute Units |
---|---|---|---|
Specialized NVIDIA cores for matrix and tensor operations | General-purpose parallel computing units in NVIDIA GPUs | Used in real-time ray tracing acceleration | Contribute to general-purpose computing tasks |
Accelerate deep learning workloads and tensor operations | Can offload CPU by splitting intensive computation workload | Perform ray-tracing calculations | Execute general-purpose instructions |
Limited contribution in graphics rendering and tasks which does not involve matrix manipulations | Can contribute to general-purpose GPU (GPGPU) computing | Used to comprehend light interactions in games with accuracy | Can be found in various processing units like GPUs and CPUs |
First introduced in Turing architecture | Found in several architectures, including Turing, Pascal, and Kepler | Enhance reflections, shadows, and global illumination in games | Process multiple tasks in parallel |
DLSS Tensor Cores
DLSS (Deep Learning Super Sampling) is a technology developed by NVIDIA that utilizes tensor cores to provide real-time AI-powered upscaling and image reconstruction. Through this upscaling, the modern GPUs can produce additional frames from the existing ones. Therefore, through the DLSS technology, a significant gain can be obtained in the FPS count. It is designed for gaming applications to enhance visual quality and performance[3].
DLSS leverages machine learning algorithms to upscale lower-resolution images in real-time, rendering games at a lower resolution while still achieving high-quality visuals. As a result, this can help improve performance by reducing the computational load on the GPU. Therefore, tensor cores play a crucial role in this DLSS process by accelerating the deep learning computations required for image upscaling and reconstruction[4].
GPU Utilization With Tensor Cores
Tensor cores are specialized cores that do not contribute to general computing and graphics processing tasks. However, there are some ways in which these cores can be utilized to improve performance in areas other than deep learning and specialized matrix tasks. Some of these ways are:
- Workload optimization: Optimizing the workload and algorithms specifically for these specialized hardware units is important to maximize GPU utilization with tensor cores. This involves utilizing libraries, such as NVIDIA’s CUDA and cuDNN, that provide optimized functions for tensor core utilization. Implementing mixed-precision techniques and leveraging the capabilities of tensor cores for matrix operations can significantly improve GPU utilization[5].
- Precision modes: Tensor cores support various precision modes, including FP16 (half-precision), BF16 (bfloat16), and INT8 (integer precision). Choosing the appropriate precision mode based on the requirements of the deep learning model can impact GPU utilization. Reduced-precision modes like FP16 or INT8 can increase GPU utilization by allowing more operations to be processed simultaneously[6].
Tensor Cores support FP16 input and FP32 compute capabilities Credits: Nvidia - Batch size: The batch size, which refers to the number of samples or data points processed in parallel, can affect GPU utilization. Increasing the batch size can help fully utilize the available tensor cores optimized for parallel processing. However, it would be best to balance increasing the batch size with available memory resources to avoid excessive usage[7].
- Data parallelism: You can efficiently utilize tensor cores when processing data in parallel across multiple GPU cores. Implementing data parallelism techniques, such as distributing the workload across multiple GPUs or parallel processing frameworks like TensorFlow or PyTorch, can help maximize GPU utilization[8].
Final Thoughts
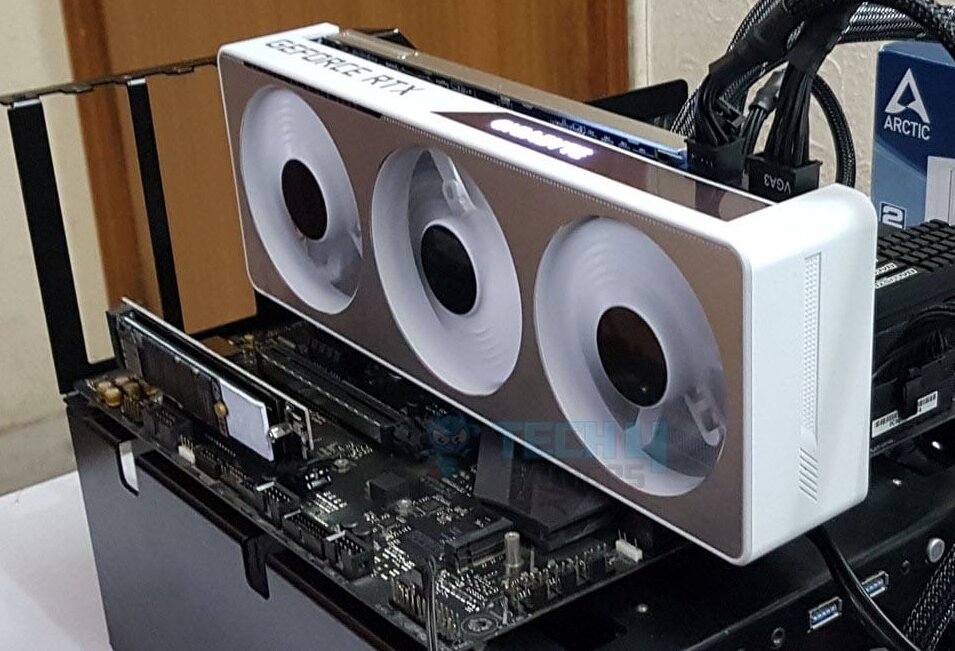
Summing up, tensor cores can accelerate deep learning workloads through quick matrix manipulations. While these cores may not directly enhance graphics performance, they are advantageous in fields where matrix manipulations are required. Moreover, an effective technique to utilize these cores in the interest of gamers is through DLSS technology. The said technology improves FPS count by generating additional frames through AI techniques.
Moving on, compute units are a general type of hardware found in various processing units, e.g., GPU and CPU. The task of computing units is to perform general computation tasks. CUDA cores are such an example of parallel compute units in NVIDIA GPUs. Additionally, it would be best to transform your general workload into specialized workloads to increase GPU utilization through tensor cores.
Related Helpful Resources By Tech4Gamers:
References:
- Oregon State University. GPU 101. Retrieved from: https://web.engr.oregonstate.edu/~mjb/cs575/Handouts/gpu101.2pp.pdf
- University of California Riverside. DEEP LEARNING VS. MACHINE LEARNING: WHAT ASPIRING DATA SCIENCE PROFESSIONALS NEED TO KNOW. Retrieved from: https://engineeringonline.ucr.edu/blog/deep-learning-vs-machine-learning/
- Song Han (Associate Professor, MIT). TinyML and Efficient Deep Learning Computing. Retrieved from: https://hanlab.mit.edu/songhan
- Deepak Narayanan (UC Berkeley). Efficient Large-Scale Language Model Training on GPU Clusters
Using Megatron-LM. Retrieved from: https://www.bu.edu/tech/files/2017/09/Intro_to_HPC.pdf - North Dakota State University. Introduction to Neural Networks. Retrieved from: https://kb.ndsu.edu/page.php?id=133762
- Justin Hensley (MIT). Hardware and Compute Abstraction Layers For Accelerated Computing
Using Graphics Hardware and Conventional CPUs. Retrieved from: https://archive.ll.mit.edu/HPEC/agendas/proc07/Day3/10_Hensley_Abstract.pdf - Louis-Philippe Morency (Carnegie Melon University). Tutorial on
Multimodal Machine Learning. Retrieved from: https://www.cs.cmu.edu/~morency/MMML-Tutorial-ACL2017.pdf - Mike Houston (Stanford University). A CLOSER LOOK AT GPUS. Retrieved from: https://graphics.stanford.edu/~kayvonf/papers/fatahalianCACM.pdf
FAQs
Tensor Cores utilize a technology developed by NVIDIA called Tensor Core Math Units (TCMU). These units work with tensor data formats and perform a mathematical operation called fused multiply-accumulate (FMA).
Tensor cores accelerate deep learning workloads by speeding up matrix operations. Modern NVIDIA GPUs contain these cores.
Whether you benefit from tensor cores depends on your application. These specialized cores can benefit you significantly if you have applications utilizing deep learning techniques. Even if you are a gamer, you can benefit from the DLSS technique that effectively utilizes these cores to improve visuals.
Thank you! Please share your positive feedback. 🔋
How could we improve this post? Please Help us. 😔
[Wiki Editor]
Ali Rashid Khan is an avid gamer, hardware enthusiast, photographer, and devoted litterateur with a period of experience spanning more than 14 years. Sporting a specialization with regards to the latest tech in flagship phones, gaming laptops, and top-of-the-line PCs, Ali is known for consistently presenting the most detailed objective perspective on all types of gaming products, ranging from the Best Motherboards, CPU Coolers, RAM kits, GPUs, and PSUs amongst numerous other peripherals. When he’s not busy writing, you’ll find Ali meddling with mechanical keyboards, indulging in vehicular racing, or professionally competing worldwide with fellow mind-sport athletes in Scrabble at an international level. Currently speaking, Ali has completed his A-Level GCEs with plans to go into either Allopathic Medicine or Business Studies, or who knows, perhaps a full-time dedicated technological journalist.